Artificial Intelligence and Machine Learning
Drug discovery is characterised by high attrition rates that add to the costly, time-consuming process of bringing new medicines to the patient. To mitigate this challenge, artificial intelligence (AI) and machine learning (ML) techniques can be employed to prioritise proposed compounds for synthesis and subsequent experimental testing. This empowers chemists to reach data-driven decisions more quickly and promotes efficient allocation of experimental resources.
We have embarked on a collaboration with the Ersilia Open Source Initiative (EOSI) to build ML models trained on the internal compound library at H3D. Combined with models trained on literature data available through the Ersilia Model Hub, these ML assets are applied to various drug discovery projects to accelerate the identification of promising compounds while lessening the costs associated with experimental research.
These predictions can be applied across the drug discovery pipeline and include screening external chemical libraries to identify hits for new drug discovery projects, ranking compounds for progression through the screening cascade, and pre-emptively flagging compounds with potential toxicity.
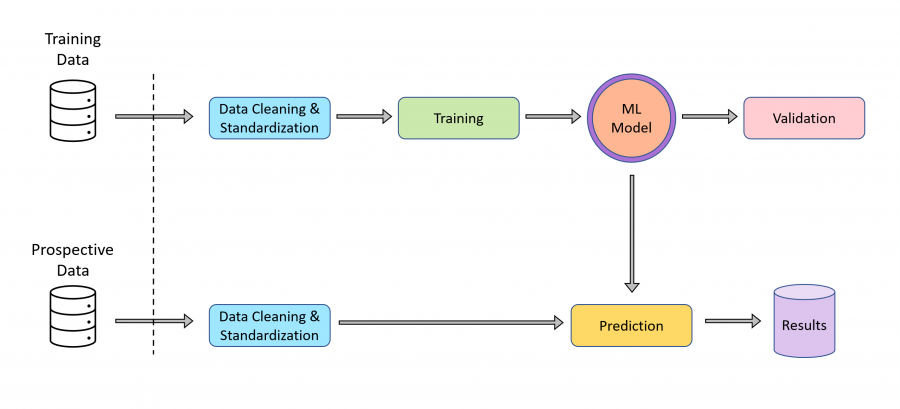
Physiologically Based Pharmacokinetic Modelling projects
The human gut microbiota constitutes a diverse group of microbial species harbouring an enormous metabolic potential, which can alter the metabolism of orally administered drugs leading to individual variation in drug responses. Pharmacomicrobiomics studies have demonstrated that human gut microorganisms and their enzymatic products can affect the bioavailability, clinical efficacy and toxicity of a wide array of drugs through direct and indirect mechanisms. The current project seeks to evaluate the influence of the microbiome on the pharmacokinetics of drugs commonly used in African populations by developing Physiologically Based Pharmacokinetic (PBPK) Models. In the first phase, the PBPK and Artificial Intelligence/Machine Learning (AI/ML) techniques are used to inform the experimental design of the Mycobacterium tuberculosis (MTB) mediated drug metabolism. This will facilitate the development of MTB mediated drug clearance. In the second phase, the gut microbiome mediated drug clearance model will be developed and integrated into the PBPK model. The input data in the PBPK models will be gathered from literature and experiments. The impact of MTB and the gut microbiome on drug pharmacokinetics will be evaluated by predicting plasma exposure of drugs using PBPK modelling. This will eventually aid in the designing of tailored doses in African populations.
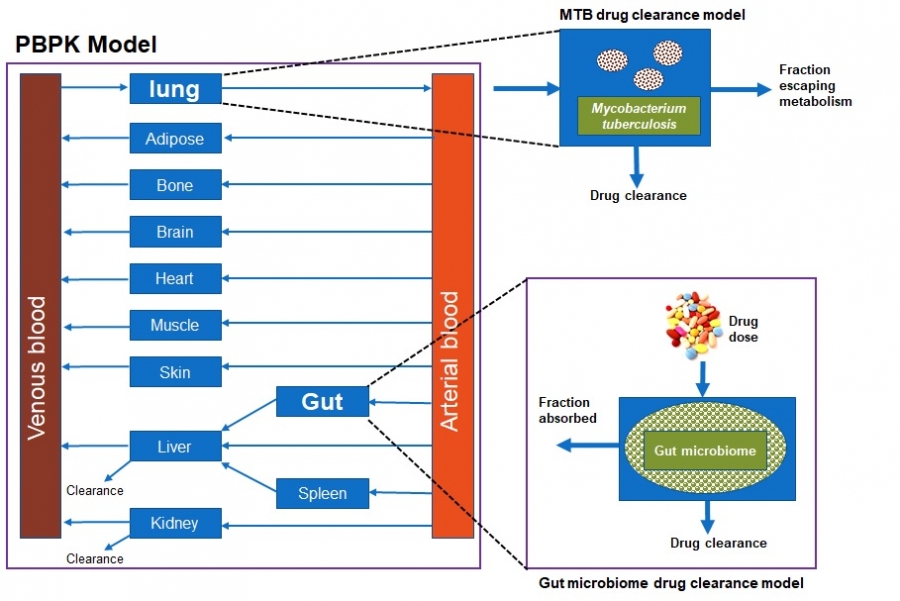